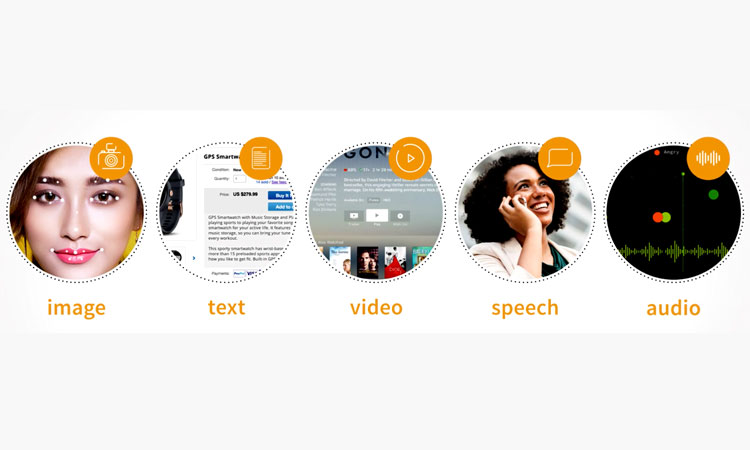
Quality
Training data accuracy and quality are critical to the success of a machine learning solution. The quality of your annotated data can decide your project’s fate, no matter how well-funded it might be. A huge advantage of outsourcing data annotation is that professional teams like Appen feature skilled, experienced professionals who work much faster and more accurately than most internally resourced teams. They have access to instructional guidelines and purpose-built tools for data annotation — and they are accustomed to processing large volumes of data. This means they can ensure a high level of accuracy, while maintaining the speed and productivity your project requires to complete on deadline. Appen trains and tests its crowd workers before they are even assigned a task, and has multiple quality checks and controls built into both the workforce management processes and data annotation platform. This helps ensure the highest level of data quality.Scale
ML projects typically require thousands or even millions of labeled training items to be successful. While the goals of machine learning projects can vary widely in complexity, they all share a common requirement: a large volume of high-quality data to train the model. Most companies simply don’t have the existing resources to staff for large-scale data annotation projects, and it’s expensive to pull engineers and other team members off of their core work on your product to perform data labeling tasks. To cover the spread of data your system might encounter in the real world, outsourcing can provide a large, on-demand staff of qualified workers to perform these tasks. And because unique requirements can emerge as a data annotation project progresses, the ability to adapt and scale up without losing data quality is critical. Internally resourced annotation teams may not have the required experience or bandwidth to handle large amounts of data or shifting project needs. Appen’s team is accustomed to annotating huge volumes of data, and rapidly responding to requests for more or different types of data and metadata. With Appen’s global resources, we can also help extend your product globally, localizing it for new markets using data from in-market annotators — native speakers with a grasp of local cultural nuance. This is an important aspect of projects involving language-based products, for example. Appen boasts a global crowd of over 1 million annotation professionals who can address this very issue.Speed
Relying on an internal team for annotation might delay the completion of your project, as these employees already have full-time obligations to attend to in addition to annotating hundreds of images. There will also be some training and ramping-up with these employees, and that can take time. If your project lacks urgency, slower time-to-completion might be acceptable, but many companies with ML projects feel pressure to get a product to market before competitors beat them to the punch. Outsourcing your annotation project to a highly trained, dedicated team can mean the difference between weeks and months. Another benefit of outsourcing is that the service can rapidly recruit data annotators with specific requirements — such as native speakers for a target demographic — and can easily ramp up and ramp down the crowd of annotation workers as project needs fluctuate. By outsourcing to a vendor that takes a managed services approach like Appen, everything from consulting to annotation task design to workforce management to quality assurance is handled externally, with repeatable processes.
Mitigating internal bias
We’ve addressed training data bias in more detail in previous blog posts, but mitigating internal bias is one of the biggest benefits of outsourcing your annotation project.. Bias in machine learning creates results that are systematically prejudiced due to faulty assumptions. When this occurs, the accuracy of your annotated data suffers, and so does your end solution. It’s worth briefly running through three of the most common causes of bias in machine learning training data:
- Sample bias occurs when the data you use to train your model doesn’t accurately represent the environment that the model will operate in. While no data set is going to represent the real world with 100% accuracy, companies like Appen can help develop the most appropriate training data for your project.
- Prejudice bias results from training data that is influenced by cultural or other stereotypes during the annotation process. Appen has specific protocols in place and employs thousands of diverse, highly skilled annotation professionals from all over the world to mitigate this exact issue.
- Internal bias happens when internal team members have a preconceived expectation of the way a given model might behave and, as a result, unconsciously provide annotation data with a given outcome in mind.