Best Practices for Deploying Successful AI
If you aren’t working with artificial intelligence, you will be soon. We interact with AI nearly every day, forcing many companies to experiment in the space. No matter where you’re at, you will likely encountered some challenges along the way. Two of the more complex elements for successfully implementing AI within your business are data acquisition and governance. There are several best practices that can help direct you on how best to build and deploy AI solutions that work. Setting yourself up for long-term success will ultimately require you to build comprehensive AI governance frameworks (especially around data governance), and scalable data pipelines. We’ll break down the key considerations for AI governance and a step-by-step guide to training data pipeline creation and maintenance.Defining AI Governance
AI governance is the framework that oversees an organization’s AI usage and implementation. How each organization defines this framework is influenced by their industry, internal corporate rules, regulations, as well as local laws. In any case, there’s no one-size-fits-all approach; each organization should choose what suits its needs best. Generally, though, there are three key areas of AI governance that frequently appear in frameworks:Performance
How you measure your model’s performance is an important factor in development. Your team should develop a series of metrics that you’ll track from initial model build and post-deployment to ensure the model performs (continues to perform) as expected. There are a couple of critical factors to incorporate into your metrics: Accuracy On the one hand, when it comes to accuracy you want to consider the precision and recall of your model. Is it meeting your desired confidence thresholds when making predictions? If not, you’ll need to iterate. On the other hand, you’ll want to consider whether your model has all of the context it requires to make accurate predictions. Your data will give you the answer here, but ensure it includes all of your use cases and known edge cases. Bias/Fairness Incorporate metrics that measure bias in your model’s performance. There are third-party tools available that can help track this. Bias can come from sampling—i.e., how you collected the data, from where, and by whom—and also from who you have annotating your data. For example, top facial recognition softwares have been shown to have greater error rates for darker-skinned individuals than lighter-skinned. Black women, for instance, see error rates over 25% versus just 1% for white men. This is a problem of the data collected (under-representing people of color) and who labeled the data (mostly white men), as their lack of diversity reflected poorly in the final solution. There are best practices you can implement in your AI data acquisition and governance frameworks to reduce bias in AI.Transparency
Your organization may be subject to legislation that requires you to show how your AI model reaches a decision. The General Data Protection Regulation, or GDPR, is one such example in Europe that empowers consumers with rights to transparency. Even if you’re not subject to regulation, the explainability of your AI models is still critical for both your end-users and reproducibility. As you build your model, thoroughly document how it works. Your governance framework can address your documentation practices and level of commitment to transparency.Ethics
Ethics is the third area that’s very common to find in an AI governance framework. Ethics play a role throughout AI implementations, starting with ensuring the intent of the solution is ethical and ending with whether the model continues to perform as intended. In this section, you’ll want to define what responsible AI looks like from pilot to production to your organization and what kind of processes you’ll have in place to ensure those requirements are met.Data Governance: Areas to Address
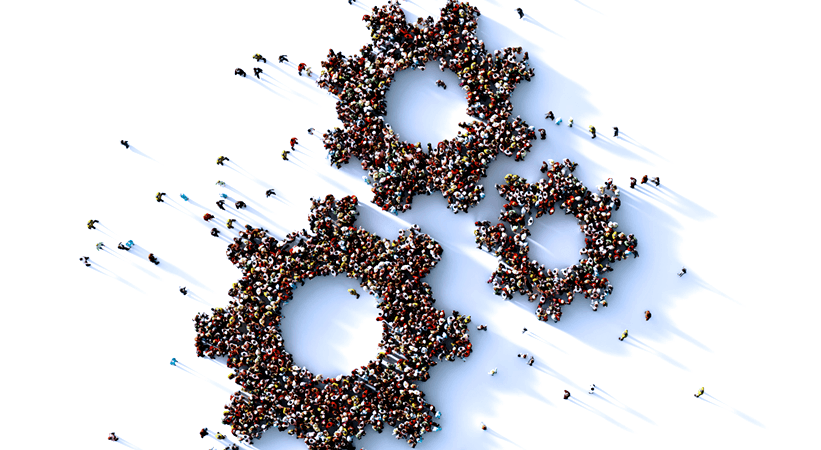
Availability
Your data is accessible and consumable by those who need it. This section should answer the question of who in your organization can see what.Usability
Your data is structured, labeled, and easy to use. Data scientists spend large amounts of time wrangling data to make it usable. To reduce this time, have data pipelines and processes in place that make data preparation faster, easier, and more scalable.Integrity
Your data maintains its structure, qualities, and completeness across its lifecycle. Your data pipeline should center on ensuring the data you use is consistent throughout your model build process.Security
Your data is protected from corruption, unauthorized use, or modification across its lifecycle. The data used for AI can often include personal information. Have security checks in place that are appropriate for the type of data you’re using, especially if that information is sensitive. Learn more about AI and data protection regulations and certifications that you should be aware of or think about when outsourcing data collection and annotation.Training Data Pipeline and Maintenance
As we refer repeatedly to data pipelines, it’s helpful to know best practices for building and maintaining these processes. Let’s walk through a full data pipeline from start to finish:1. Data Acquisition
You’ll collect data from one or a variety of sources. These may include internal sourcing, readily-available data, open-source datasets, or third-party vendors. The goal is to source data that covers all possible use cases and edge cases for your end-users. Be sure you’re sourcing your data ethically.2. Data Annotation
In the next step of your data pipeline, you’ll perform data annotation (e.g., image classification, audio transcription, or other types). Who you select to label your data is very important; these people need to have diverse backgrounds and perspectives so as to reduce the potential for bias. For large annotation jobs, companies often rely on third-party crowd workers sourced from around the globe.3. Data Auditing
While you should audit your data at each stage in the process, after annotation it’s especially important to ensure your data labels are accurate and unbiased. Annotations should account for all use cases. Once you’ve performed a data audit and found that your labeled data meets your accuracy criteria, you’re ready to train your model and deploy it.4. Model Updating
Very few use cases rely on static models. In most scenarios, you need to update your model frequently to reflect the real world and changing data. Your data pipeline should continue to serve you long after deployment as you continue to create new training data to avoid model drift or stagnancy. This component of model maintenance is often underlooked but is mission-critical to achieving long-term success in AI. We break down what a comprehensive data pipeline for autonomous vehicles might look like, as an example.In Summary: AI Best Practices
If anything should be clear, it’s that AI data acquisition and governance frameworks are fundamental to building your organization’s AI strategy. Beyond these elements, there are naturally many more questions your team will need to answer throughout the model build process. At a high level, these questions often touch on areas like:- Know the problem. Can your problem be solved by AI?
- Understand the data. Do you have all the data you need to train an AI algorithm?
- Determine key metrics. Which metrics around accuracy, efficiency, cost savings, bias, etc. indicate success for your model?
- Audit performance. Do you have ways of identifying model drift?
- Iterate. Are you consistently retraining and tuning your model, even after deployment?