Ethical AI Techniques to Minimize Bias Throughout the Model Build Process
Artificial intelligence ethics are often thought of in the context of the model itself. Meaning, the focus is on the ethical quality of a model’s prediction and outcome, as well as the impact it has. Even though most companies focus on reducing bias in their models, that’s not enough to say that ethical AI techniques are in place. When it comes to responsible AI, we need to factor in the ecosystem as a whole. It’s critical to think about the entire journey when it comes to implementing ethical AI: what is the AI model built on, what does the AI model do, and what will this model impact? These are just some of the big questions that companies need to ask. And the reality is, the answers to many of these questions aren’t limited to AI, but are just responsible practices by good corporate citizens. Despite the tough questions, there’s good news. Real World AI that’s built responsibly is often more successful.Four Key AI Ethics Considerations
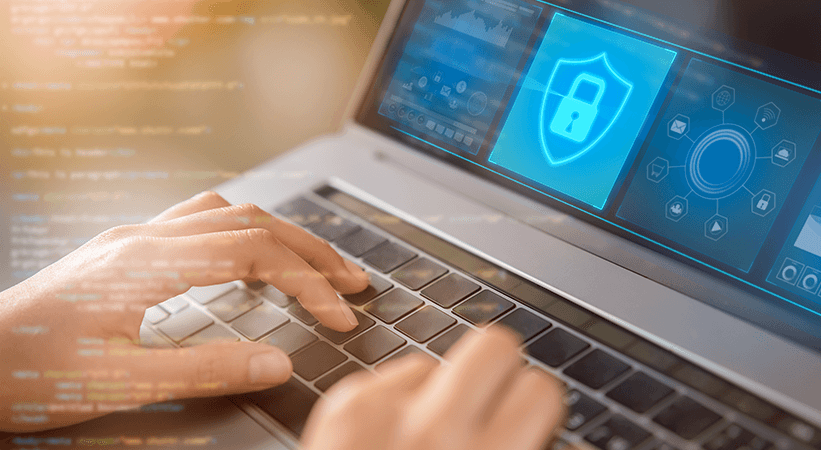