What’s the paper about?
It’s well-known that crowdsourcing can produce a high rate of noisy and low-quality data. While techniques such as “golden answers” help filter noise for certain kinds of data collection tasks, these techniques are difficult to use with utterance collection due to its open-ended nature, which results in large amounts of data that must be discarded. This means that obtaining high-quality utterance data often requires careful design and multiple iterations of the crowdsourcing task, which can lead to a significant increase in costs. In her paper, Liu considered several variations of commonly used workflows for crowdsourced utterance collection and attempted to determine how they affect data quality. Two cost-saving strategies were examined closely: Using a two-tier payment scheme to incentivize crowd workers and using automatic verification of the input utterance data. Three defining questions emerged at the outset of the project:
- How much does utterance quality improve when using two-tier payment, compared with paying workers in full up front?
- Since a majority of the cost lies in human verification, how does data quality improve using automated verification as compared to using no automatic verification?
- Is there a strategy that can be used as a stopping criterion for data collection without impacting model performance?
Does a two-tier payment approach deliver better results?
Liu’s team performed an experiment to evaluate whether paying the workers upfront versus offering a two-tier payment would improve utterance data quality. For the single-tier approach, they paid the total cost upfront. For the two-tier condition, they paid one third of the cost upfront, followed by the rest upon successful validation of an utterance.Can automated verification achieve a human level of accuracy?
The team investigated whether invalid utterances could be prevented during the data collection phase, thus mitigating the cost of human verification. To do so, they created an automated text validator (or smart validator) that prevents workers from submitting gibberish and non-target language utterances. This validator estimates how likely it is to generate an utterance based on the character to character transitions of that utterance. An utterance is marked as gibberish when it has low probability.Results: Individual and combined strategies
For the two-tier payment with smart validator approach, the team expected that workers would have been motivated by the payment structure and naturally wanted to perform well, resulting in similar utterance quality as compared to a two-tier approach without automated validation. However, the increase in utterance quality in two-tier with automated validation led them to speculate that some workers who initially were not attentive realized that they needed to be more attentive after the smart validator prevented them from submission.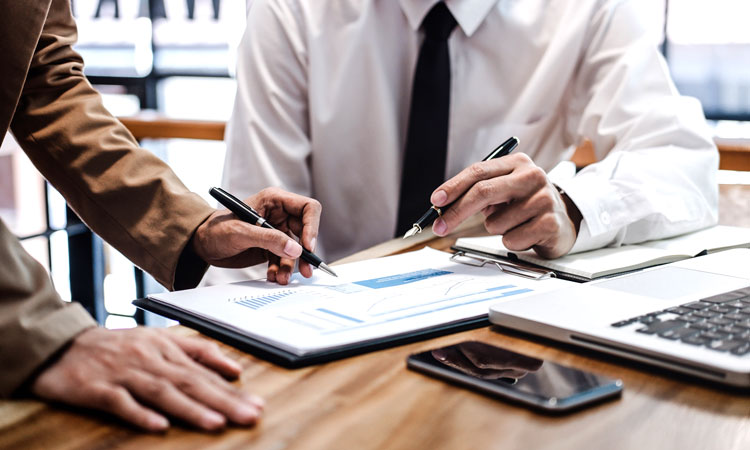
Quick takeaway: Smart validation approaches human performance to the extent that it would be a suitable cost-reduction strategy for projects that can afford slightly reduced accuracy.
Will an adaptive data collection strategy pay off?
Given the same amount of training data, different intents may reach different coverage due to the difference in the number of phrasing variations. This prompted the team to wonder whether it could be beneficial to use coverage to adaptively terminate data collection for each intent. The idea is that, rather than the common practice of crowdsourcing a consistent number of utterance examples for an intent (a “fixed strategy” for a specific application), we can stop collecting data when the coverage of that intent exceeds a threshold (an “adaptive strategy”).Quick takeaway: For the purposes of this case study, Liu’s team found that employing an adaptive strategy was 40% more cost-efficient compared to a fixed strategy.
Conclusion
Training data is the key to building machine learning models and finding the most cost-effective way to collect training data via crowdsourcing still remains an open question. Liu’s findings demonstrated that using a smart validator can significantly increase data quality with no increase in cost. Though human verification was found to be slightly more effective than the smart validator, it also increased the cost substantially. The paper also demonstrated that using the metric of coverage can be a cost-effective way to adaptively terminate the data collection process while maintaining required model performance.The final takeaway? Liu’s team has provided clear guidance for future data practitioners working with automated language systems: It’s possible to reduce costs without sacrificing data quality.— At Appen, we’ve helped leaders in machine learning and AI scale their programs from proof of concept to production. Contact us to learn more.