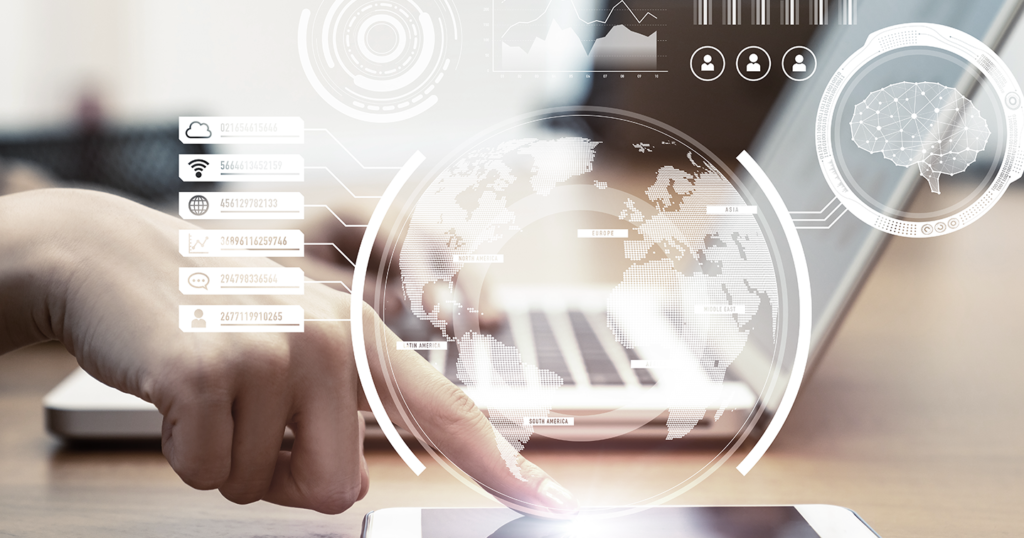
1. High-quality Data Remains a Major Roadblock for AI
The majority of AI projects still don’t make it to production, proving that companies still face significant challenges in implementing AI successfully. One of the largest hurdles remains obtaining high-quality data. In a recent survey, respondents cited the lack of skilled individuals and the lack of data (or data quality issues) as the top two bottlenecks for launching AI. This isn’t surprising: most of the time data scientists spend on AI projects is spent on collecting and preparing data, and the quality of that data has a tremendous impact on how well the model performs. Getting this part right is critical, but resource-intensive. Any company that solves the data challenge will unlock major competitive opportunities. Indeed, companies are exploring expanded solutions, such as hiring external data providers to access needed expertise and tooling (this according to the latest State of AI report).2. AI Use Cases are Becoming Narrower
Selecting a narrow business problem to solve is a top tip for building high-performing AI solutions. It seems that companies are starting to learn that this is a critical first step, and are choosing to be more specific and less broad in the problems they choose to focus on. To illustrate this, here are a few projects Appen has recently worked on with a very narrow focus: Biz-speak: The company was building an AI model that would suggest improvements or alternative phrases to common “biz-speak” (or business jargon). These terms were often very nuanced, presenting us with a challenge of capturing sufficient data. Body movements: A company working on a model to automate personal training faced an obstacle: movement profile changes with age. To help solve this, they asked us to capture and annotate video of seniors doing somersaults. Long-tail languages: Covid-19 information needs to be shared globally, but the problem is that translation technology doesn’t support all languages. We were tasked on a project to collect and annotate data for languages that are very uncommon, such as Dari, Dinka, Hausa, and more. These examples demonstrate how companies are narrowing their focus to very specific use cases, which has implications both for how training data is being used and what type of data is being collected.3. Shift from Model-centric to Data-centric AI
Is it better to improve code or training data? It’s a question that was at the forefront of AI minds in the past several years. Several famous experiments demonstrate that the magic is in the data, and we’re seeing a shift from model-centric development to data-centric. With model-centric AI, the idea is to use available data and develop models that compensate for any noise and inaccuracies. Data-centric, on the other hand, focuses on improving the volume or quality of the data. A well-known AI practitioner conducted an experiment using a computer vision model that detects defects in steel sheets. He split his team into two and had one group work on improving the code of the model only, and the other group work on improving the data given to the model. He discovered that improving the code had virtually no impact on the performance of the model, while improving data provided a huge uplift (from 76% to 93%). With data improvement alone, the model was even able to beat humans at spotting defects, who only had a 90% accuracy rate.4. Emerging Need for Training Data Operations
As companies acknowledge the importance of training data to the success of their AI models, naturally there comes a growing need for ways of managing training data. After all, developing training data includes many tasks: data collection, ingestion, exploration, labeling, validation, and preparation. Having a governance framework for these items will prove a useful tool for AI teams going forward. A functional data governance framework may include the following key features:- Version control for traceability
- Data security protocols
- Access controls
- Data pipeline monitoring
- Collaboration protocols