How Artificial Intelligence Gives OCR a Boost
Artificial intelligence is transforming the capabilities of optical character recognition (OCR) tools. An area of computer vision, OCR processes images of text and converts that text into machine-readable forms. In other words, it takes handwritten or typed text within physical documents and converts them into digital formats. In the 1990s, many business owners used OCR, sometimes called text recognition, to convert physical documents into digital files. Since then, the quality of OCR technology has improved, but demand has increased for broader usability. Recent developments with AI have amplified OCR’s utility thanks to higher accuracy and greater speed. With the benefit of AI, human supervision isn’t needed at every step.OCR and AI: A Benefit to Businesses
Before the invention of OCR, converting physical text to digital was a manual effort: a person would have to retype each document, a time-consuming task prone to mistakes. With OCR, the conversion happens quickly and with greater fidelity to the original content. Once OCR converts a hard copy into its digital form, viewers can edit, format, and search the document. They can also send it easily via email, include it in a website, and store it in compressed files. Naturally, this eliminates the need for physical storage space, a cost-savings for businesses that heavily rely on documentation, such as mortgage brokers or legal firms. As teams combine OCR with AI and machine learning (ML) techniques, they’re able to use machines to more accurately convert text and check for errors that may occur during the conversion. AI can better interpret handwriting as well, opening up opportunities for digitizing a wider range of documents. Handwriting still presents a challenge to AI due to the uniqueness of each individual, but with more handwriting training data, machines are gaining greater ability on that front as well. As an example of AI-powered OCR, imagine an OCR tool was converting print invoices into digital copies. Let’s say the scanner identified the invoice total as $500, when it was really $5,000. Before AI, the OCR tool wouldn’t pick up on this mistake and it would be up to human review to catch it. With AI tools, however, an algorithm can review the entire document, calculate that the subtotals for services provided should add up to $5,000, and fix the error without a human needing to supervise. This document comprehension capability helps businesses analyze numerous documents without committing human labor to the task. Reducing tedious administrative work can be critical to maximizing employee engagement and reducing turnover. Researchers expect demand in AI-powered OCR to continue as these tools become more efficient and cost-effective.How OCR Works
An OCR system features a combination of hardware and software. The system’s goal is to scan the text of a physical document and translate the characters within that document to a code that’s then used for data processing. Think of this in context of postal and mail sorting services – OCR is core to their ability to operate quickly in processing destination and return addresses to sort mail faster and more effectively. The system does this in three steps:1. Image Pre-processing
In step one, the hardware (usually an optical scanner) processes the physical form of the document into an image – such as an image of an envelope. The goal of this step is for the machine to be accurate in its rendition, but also to remove any unwanted distortions. The resulting image is converted to a black and white version, which is then analyzed for light areas (background) versus dark areas (characters). The OCR system may also categorize the image into separate elements if needed, such as tables, text, or inset imagery.2. Intelligent Character Recognition
AI analyzes the dark areas of the image to identify letters and numbers. Typically, AI targets one character, word, or block of text at a time using one of the following methods:- Pattern recognition: Teams train the AI algorithm on a variety of text, text formats, and handwriting. The algorithm compares the characters on the scanned envelope image to the characters it has already learned in order to identify matches.
- Feature extraction: To recognize new characters, the algorithm applies rules regarding specific character features. Features may include the number of angled, crossed, or horizontal lines and curves in a character. An “H” for example has two vertical lines and one horizontal in between; the machine will use those feature identifiers to identify all “H”s on the envelope.
3. Post-processing
In step three, AI corrects errors in the resulting file. One method is to train the AI on a specific lexicon of words that will be found in the document. Restrict the AI’s output to only those words/format to ensure no interpretations fall outside of the lexicon.Applications of OCR
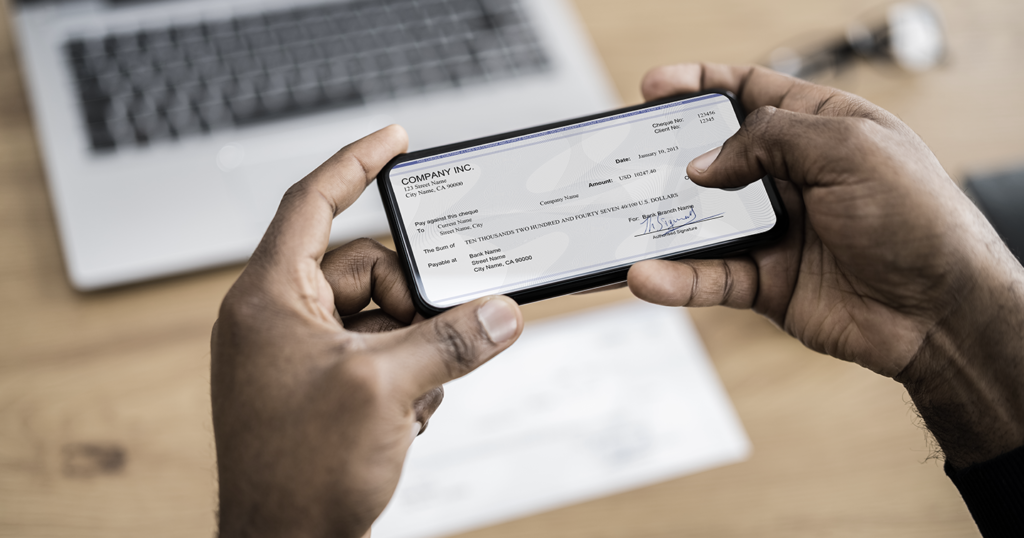
Word Processing
Perhaps one of the earliest and most common uses for OCR is word processing. Users can scan printed documents to be converted into editable and searchable versions. AI helps to ensure these documents are converted with the greatest accuracy possible.Legal Documentation
OCR can place important signed legal documents, such as loan paperwork, into an electronic database for easy reference. Multiple parties can easily view and share the documents as well.Retail
Retailers use serial numbers to represent their products. In retail outlets or warehouses, robots can scan product barcodes, apply OCR to extract the serial numbers from these barcodes, and use that information to track stock.Historic Preservation
OCR turns historic documents into searchable PDFs. This is especially helpful for archiving old newspapers, magazines, letters, and other historical records.Banking
Today, you can use your smartphone to take a front and back photo of a check you’d like to deposit. AI-powered OCR technology can automatically review the check to confirm its validity and that it matches the amount you’re looking to deposit. OCR technology would not be as advanced today without a boost from AI. AI paired with OCR reduces errors, dramatically improves conversion accuracy, and provides additional analyses to documents. The reduced administrative and cost burden is a major draw for companies looking to secure a more efficient method of managing documents.Insight from Kirsten Gokay, an Appen Expert on Optical Character Recognition
At Appen, we rely on our team of experts to help you build cutting-edge models utilizing OCR. Kirsten Gokay, Senior Product Manager at Appen, works to ensure Appen customer models using OCR are executed successfully. Kirsten’s top three insights on utilizing optical character recognition include:- Use the right data for your model, ensuring that it maps to the type of data you expect to see in the real world. For example, if you’re training a model to automatically transcribe receipts, your data should consist of receipts containing the values you’re looking for. Your data should also be well-rounded: images at different angles, different types of image quality, so on and so forth – particularly if this model will be applied to user-generated content.
- The right tooling matters! Because your training data needs to be well-rounded, the tool you use to annotate the data must be able to work with all manner of documents.
- A Human-in-the-Loop approach is crucial for success. To ensure the accuracy of your model, it’s best not to rely on AI alone. Bringing people into the annotation process allows you to find and correct errors before training.