What You Need to Know About Quality Assurance for Your AI Models
Launching an artificial intelligence (AI) model that’s accurate, reliable, and unbiased is undeniably a challenge. Organizations that manage to achieve success in AI initiatives are likely aware that the quality assurance (QA) process is very different with AI than traditional QA processes. Quality assurance plays a critical role in the accuracy of an AI model and shouldn’t be overlooked. Any company hoping to deploy effective AI must build in QA checks throughout their model’s lifecycle. We often discuss the five phases of building world-class AI, which include:- Pilot
- Data Annotation
- Test & Validate
- Scaled Deployment to Production
- Retraining
Phases 1 and 2: Pilot and Data Annotation
This is when businesses should be identifying the problem they’re going to solve and gathering the data. QA confirms the data used for model training is of sufficient quality.Phases 3 and 4: Test & Validate and Scaling
During these phases, the model is built and is being tested and tuned as it scales to a wider and wider audience. QA is vital during these phases as it verifies the built model that goes live is of sufficient quality – especially as the model runs off real data, not test data.Phases 5: Retrain
Regular retraining is critical for almost every AI model. QA confirms the model continues to deliver sufficient quality when running and offers the opportunity to continue to improve accuracy. Several QA steps require a check comparing a metric of your data or model to a predefined value or threshold. Others are analyses or reviews, requiring time, manual effort, domain knowledge, and common sense. In any case, building in QA checks and balances is an integral component of deploying successful AI.Quality Assurance and Training Data
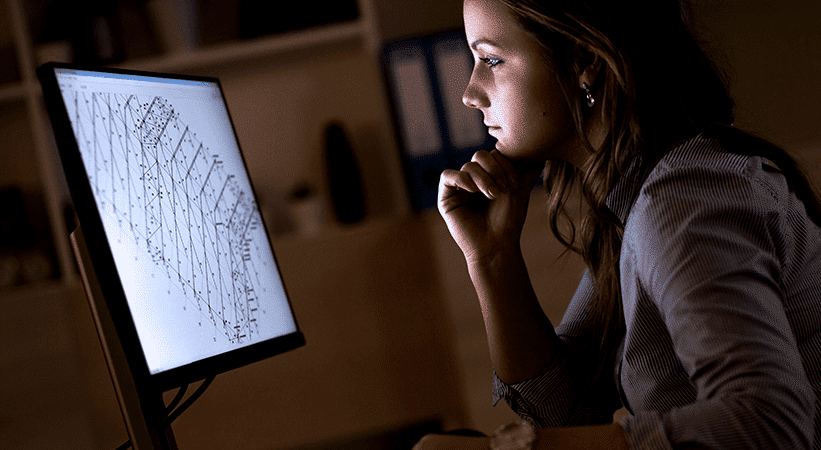