Everything You Need to Know About Text Annotation with Yao Xu
Every day, we interact with different media (such as text, audio, images, and video), relying on our brain to process what media we are seeing and make meaning out of it to influence what we do. One of the most common types of media is text, which makes up the languages we use to communicate. Because it is so commonly used, text annotation needs to be done with accuracy and comprehensiveness. With machine learning (ML), machines are taught how to read, understand, analyze, and produce text in a valuable way for technological interactions with humans. Per the 2020 State of AI and Machine Learning report, 70% of companies reported that text is a type of data they use as part of their AI solutions. Understandably so, as the cost-savings and revenue-generating implications of text-based solutions across all industries are enormous. As machines improve their ability to interpret human language, the importance of training using high-quality text data becomes increasingly indisputable. In all cases, preparing accurate training data must begin with accurate, comprehensive text annotation.What is Text Annotation?
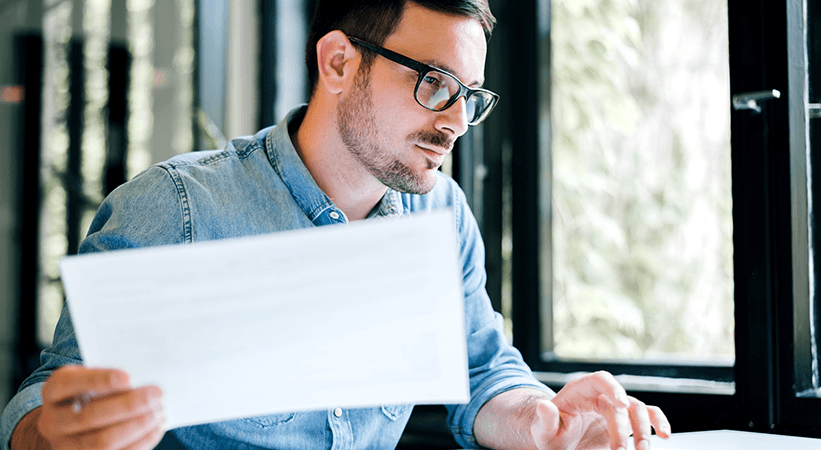
Types of Text Annotation
Annotations for text include a wide range of types, such as sentiment, intent, semantic, and relationship. These options are available across a wide array of human languages.Sentiment Annotation
Sentiment annotation evaluates attitudes and emotions behind a text by labeling that text as positive, negative, or neutral.Intent Annotation
Intent annotation analyzes the need or desire behind a text, classifying it into several categories, such as request, command, or confirmation.Semantic Annotation
Semantic annotation attaches various tags to text that reference concepts and entities, such as people, places, or topics.Relationship Annotation
Relationship annotation seeks to draw various relationships between different parts of your document. Typical tasks include dependency resolution and coreference resolution. The type of project and associated use cases will determine which text annotation technique should be selected.How is Text Annotated?
Most organizations seek out human annotators to label text data. Human annotators are especially valuable in analyzing sentiment data, as this can often be nuanced and is dependent on modern trends in slang and other uses of language. Still, large-scale text annotation and classification tools out there can help you achieve the deployment of your AI model quickly and more inexpensively. The route you take will depend on the complexity of the problem you’re trying to solve, as well as the resources and financial commitment your organization is willing to make. Refer to data labeling methods for a comprehensive look at the annotation options available to your organization.Appen’s Text Annotation Expert – Yao Xu
At Appen, we rely on our team of experts to help provide text annotation for our customers’ machine learning tools. Yao Xu, one of our product managers, helps ensure the Appen Data Annotation Platform exceeds industry standards in providing high-quality text annotation services. She came from a science and linguistic academic background, speaks three languages, and has extensively studied ML and NLP. Her top insights when evaluating and fulfilling your text annotation needs include: Know your current goal and long-term vision- What kind of data do you need
- How much data do you need and how soon
- Is your data in a specialized domain or non-English languages
- What resources do you have
- Look beyond text-based data