How AI Adds Value to Society and Business
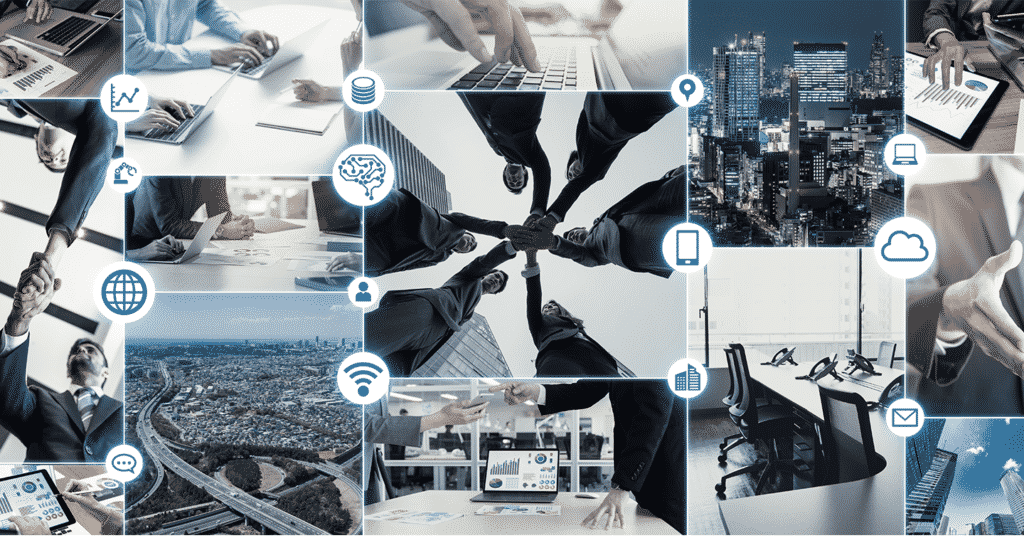
Benefits to Society
AI can improve user experience of technology, enhancing customer-to-business interactions and creating a more personalized, faster user experience. We see this in the use of fraud detection technology, personalized virtual assistants, customized search recommendations, and many other AI-powered interactions. In the best of cases, AI can even help save lives. Smart cars with driver assist capabilities are a popular example of using AI to improve road safety. But take for example the application of AI to medical imaging technology. Using ML techniques, algorithms can analyze MRIs for cancer detection. Doctors use these results to target radiotherapy more precisely, causing less damage to tissue. In some scenarios, the contributions of AI could be a matter of life or death. In another example, researchers are applying AI to extreme weather forecasts. If they’re able to predict the intensity of a tropical storm based on satellite images, for instance, they can inform rescue teams and potential evacuees of action plans before it’s too late. Major industries like healthcare, automotive, aerospace, and military are leveraging AI for safety reasons, making it an increasingly instrumental component of their operations. Ultimately, AI can have a tremendous positive impact on various areas of society, from saving time to saving lives.Benefits to Business
Organizations are turning to AI to maximize internal efficiencies as well. They’re using AI to grow their business, increase revenues, and drive down costs. In the energy sector, for example, companies use AI to predict energy consumption in advance, which helps them regulate how much production they need and generates substantial savings. Companies also use AI to optimize internal workflows. Algorithms can manage mundane tasks previously performed by humans, saving significant effort and time. A bank’s chatbot, for instance, can answer routine customer questions almost instantaneously for a speedier customer experience and less overhead for the company. AI also helps streamline machine learning processes. If a team needs to develop training data for their ML model, an algorithm can provide initial hypotheses on data labels. This makes the job of the data annotator much easier: instead of starting from scratch, they simply need to verify or correct an existing label. These algorithms are useful for providing quality control in AI development processes as well, ensuring that the labeled training data meets expectations in accuracy. In any case where a business is leveraging a lot of data, even small tweaks made to a given process can add a lot of value.Unlocking the Value of AI
Avoiding the pitfalls of AI deployment is a significant step toward tapping into the value that AI can offer your business, your customers, and in some cases society as a whole. In each stage of your model build process, it’s important to ask yourself key questions:Define a Business Problem
- What problem am I trying to solve?
- Is AI the right tool for solving this problem?
- Is there enough quality data to solve this problem with AI?
Get the Right Data
- Where will I obtain my data?
- How do I ensure my data is the right quality?
- Who will label my data and how will I ensure those labels are accurate?
- How will I reduce bias in my data?
Ensure Org Readiness
- Do I have the right team in place to implement this AI solution?
- Are key stakeholders on board with this initiative?
- Does my organization have the money, time, and people available to invest in this initiative?
Build Model
- Does my model do what I originally intended it to do?
- Is my model showing bias?
- Does my model need additional training data?
- Is my model meeting the accuracy and confidence thresholds I require?
Monitor Performance
- How will I monitor my model’s performance?
- How will I manage data drift?
- How will I scale my solution?