Building Our First Model
Because we wanted to drive revenue, when we first set out to build our AI model, our team focused on increasing purchases per session: the average number of items a buyer purchases in one user session. With that goal in mind, our AI model emphasized the sales (how many times an item was sold) over impressions (how many times an item was viewed), and less expensive items, which sold more frequently than expensive ones, were ranked higher than other items. We tried different machine learning models—models to rewrite buyers’ queries, models to generate features to be used in the ranking model, and models to rank the final search results. We then ran a series of A/B tests to assess the model results, with great success. Many of the models proved that buyer conversion had increased. Other teams were motivated by these successes and started to put in the effort to increase their purchases per session. Everything looked rosy. That is, until the finance team observed that those A/B testing wins didn’t translate into increased revenue.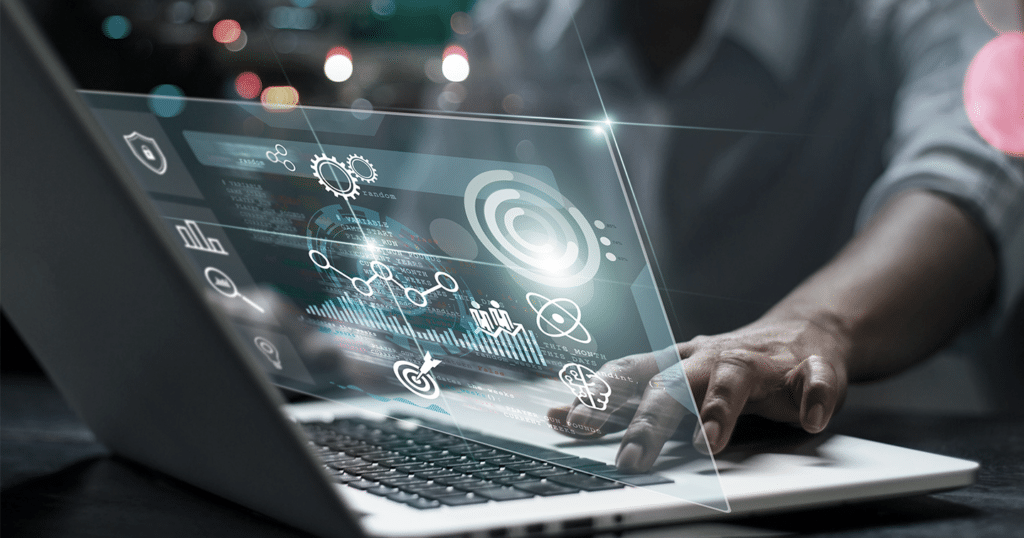