Be Persistent
Leading with AI doesn’t just happen in the blink of an eye. It’s going to take time and hard work, and it’s critical you stay persistent. Amazon, a leader in machine learning technology applications, didn’t start out using advanced AI techniques on day one. Just like everyone else, they had to go on a journey filled with discovery, successes—and the occasional course correction. For example, in 2017, Amazon launched a TV ad that accidentally triggered Alexa devices in its customers’ homes to purchase an expensive dollhouse. Not ideal, and not the best advertisement for the convenience of their machine learning devices. They stuck with progress, though, and learned from their mistakes. They were persistent, even though they started out behind the pack. Their focus on incremental progress meant that it only took five short years for Alexa to beat out Siri and Google Assistant in the smart home speaker market. Alexa may have had a slow start, but now she’s a very strong player in the market. Persistence and determination are the ingredients that build leaders in AI—not perfection from the start.Train Your Leaders to Look for AI Solutions
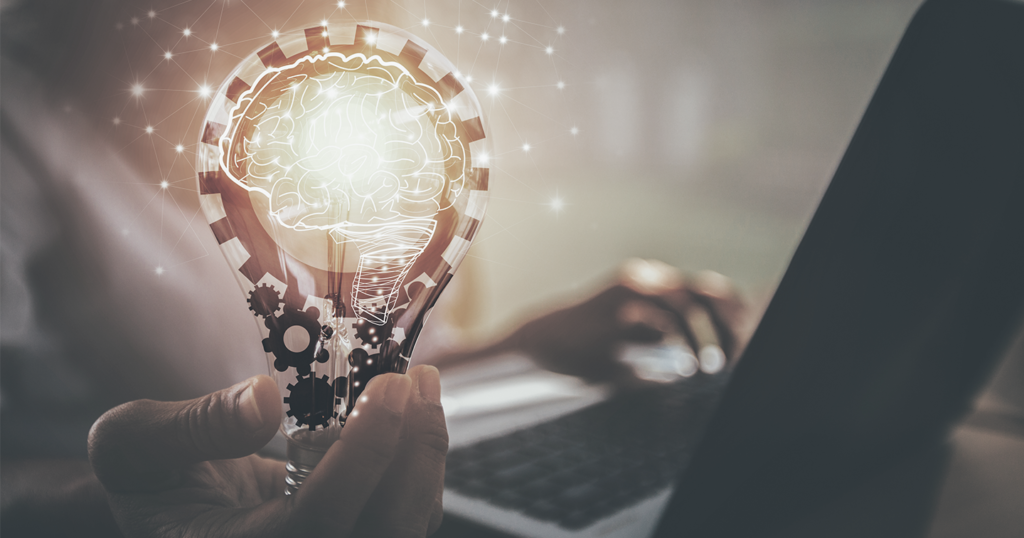