New Off-the-Shelf Datasets from Appen
Creating a high-quality dataset with the right degree of accuracy for training machine learning (ML) algorithms can be a difficult uplift for getting AI and ML projects off the ground. Not every company has a specialized team of ML PhDs, data engineers, and human annotators at their disposal. This is largely due to the expense of such a team. Instead, machine learning teams are turning to bespoke, off-the-shelf training datasets. These off-the-shelf training datasets offer a quick, cost-effective alternative, solving for both the cold-start problem as well as model improvement without the risks of collecting and annotating data from scratch. This is because these datasets that are high-quality can be used as-is or customized for specific project types. Finding datasets that have high accuracy labels can also be a difficult task. Many datasets out there may be old, uncleaned, or irrelevant. To help companies get their ML initiatives off the ground, Appen has made its entire catalog of off-the-shelf datasets available from its website. These datasets are comprised of high-quality training data to help companies ensure they know the accuracy they’re getting upfront, removing the variability out of the model training process. Users are now able to browse diverse speech and text datasets and request quotes for one or multiple datasets including:- Fully transcribed speech datasets for broadcast, call center, in-car, and telephony applications
- Pronunciation lexicons, including both general and domain-specific (e.g. names, places, natural numbers)
- Part-of-speech-tagged lexicons and thesauri
- Text corpora notated for morphological information and named entities.
Machine Learning Projects that Benefit from Off-the-Shelf Training Datasets
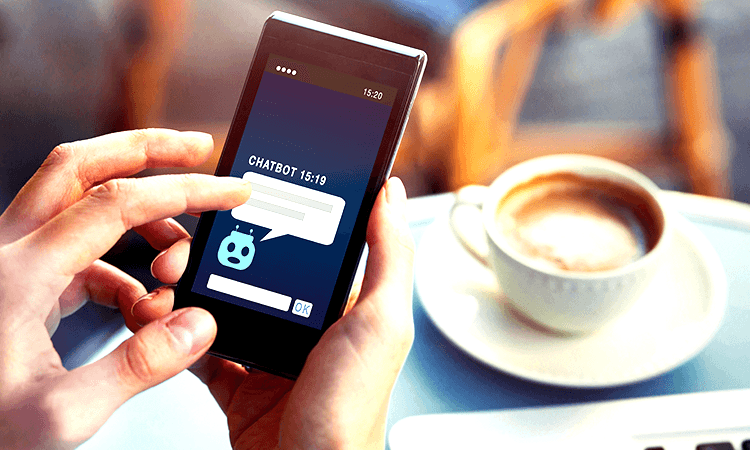