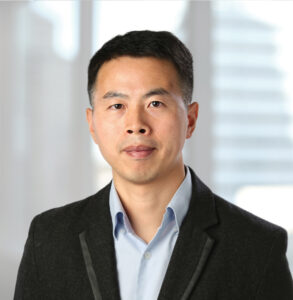
Wilson Pang | Chief Technology Officer, Appen
Wilson Pang joined Appen in November 2018 as CTO and is responsible for the company’s technology. Wilson has over 20 years’ experience in software engineering and AI. Prior to joining Appen, Wilson was Chief Data Officer of CTrip in China, the second largest online travel company in the world, where he led data engineers, analysts, data product managers, and machine learning scientists to improve user experience and increase operational efficiency that grew the business. Before that, he was senior director of engineering at eBay in California and provided leadership to various domains, including data service and solutions, search-science, marketing technology, and billing systems. He worked as an architect at IBM, prior to eBay, building technology solutions for various clients. Wilson is also the co-author of Real World AI: A Practical Guide for Responsible Machine Learning Wilson obtained his Masters and Bachelor’s degrees of Electric Engineering from Zhejiang University in China.
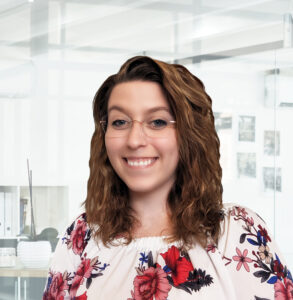
Rachel Kellam | Product Manager, Appen
Rachel is a Product Manager in Appen’s Autonomous Vehicles working group. In that role, she is working to provide high quality data on all levels of autonomy for motor vehicle clients. Prior to joining Appen, Rachel worked on data science tools to enable model interpretability, fairness testing and automated machine learning. Other passions of hers include using AI and technology to act as a catalyst towards solving humanitarian-centered problems for non-profits around the world.